Anticipate users' next move with Predictive Segmentation
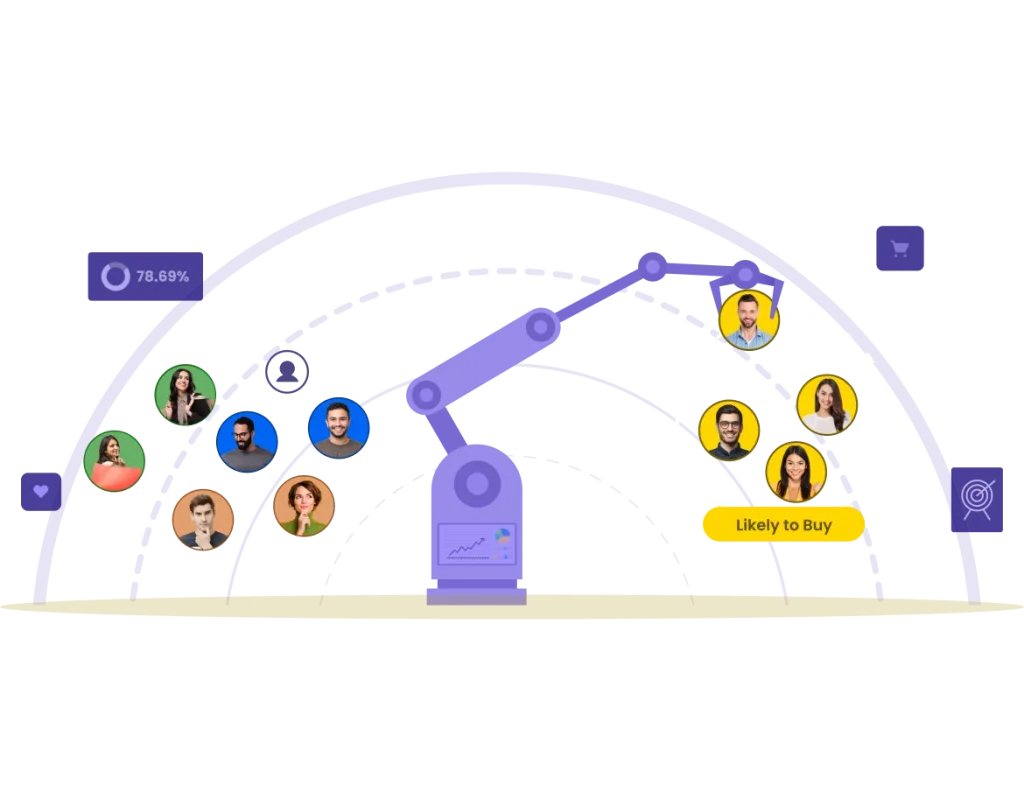
Harness machine learning to identify users
likely to take action.
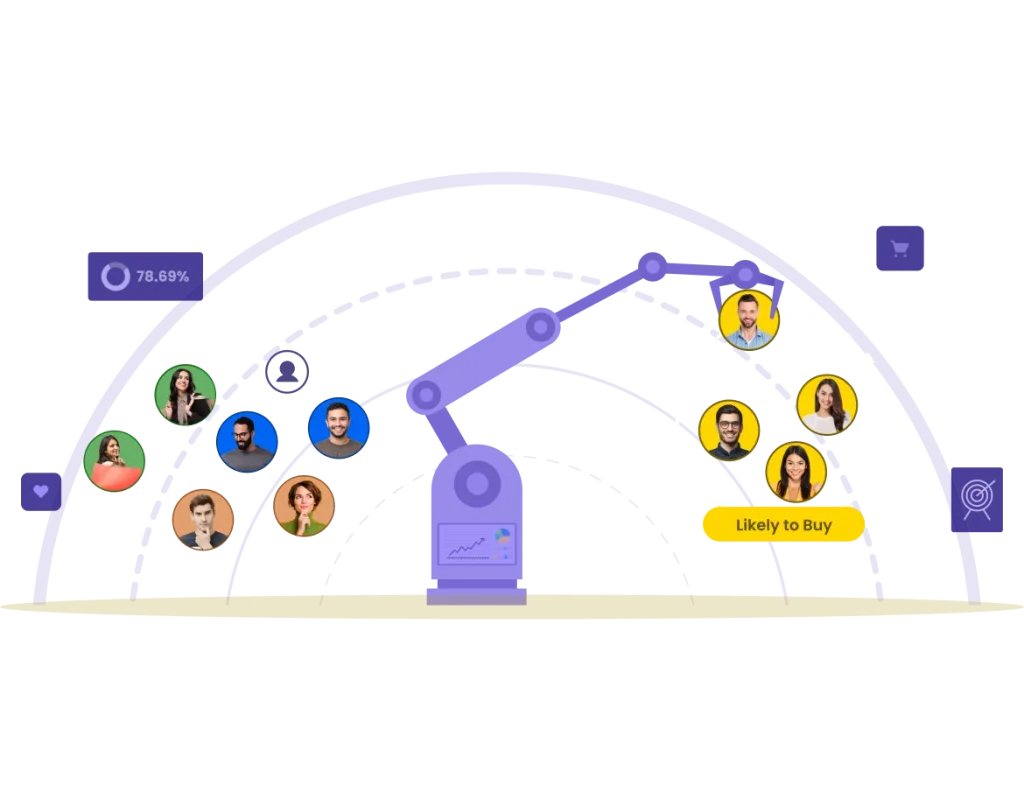
800+ global brands trust WebEngage for customer engagement and retention
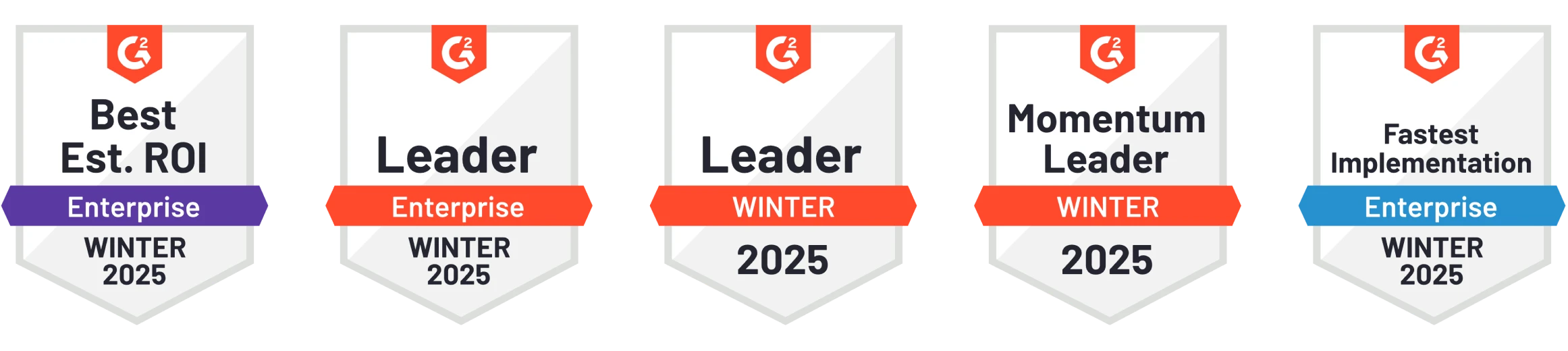
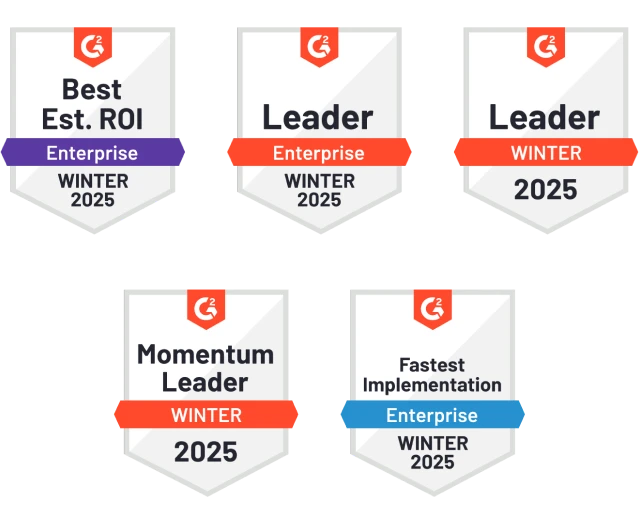
Why use Predictive
Segmentation by WebEngage?
Analyze thousands of customer touchpoints to target precisely.
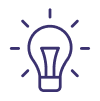
No more
Analysis Paralysis
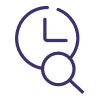
Prediction
with Precision
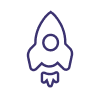
Simple to launch
campaigns
Use our Propensity Model to craft
messages that hit the mark!
Split users into Most, Moderately and Least Likely.
Use these segments as auto-refreshing lists for your campaigns!
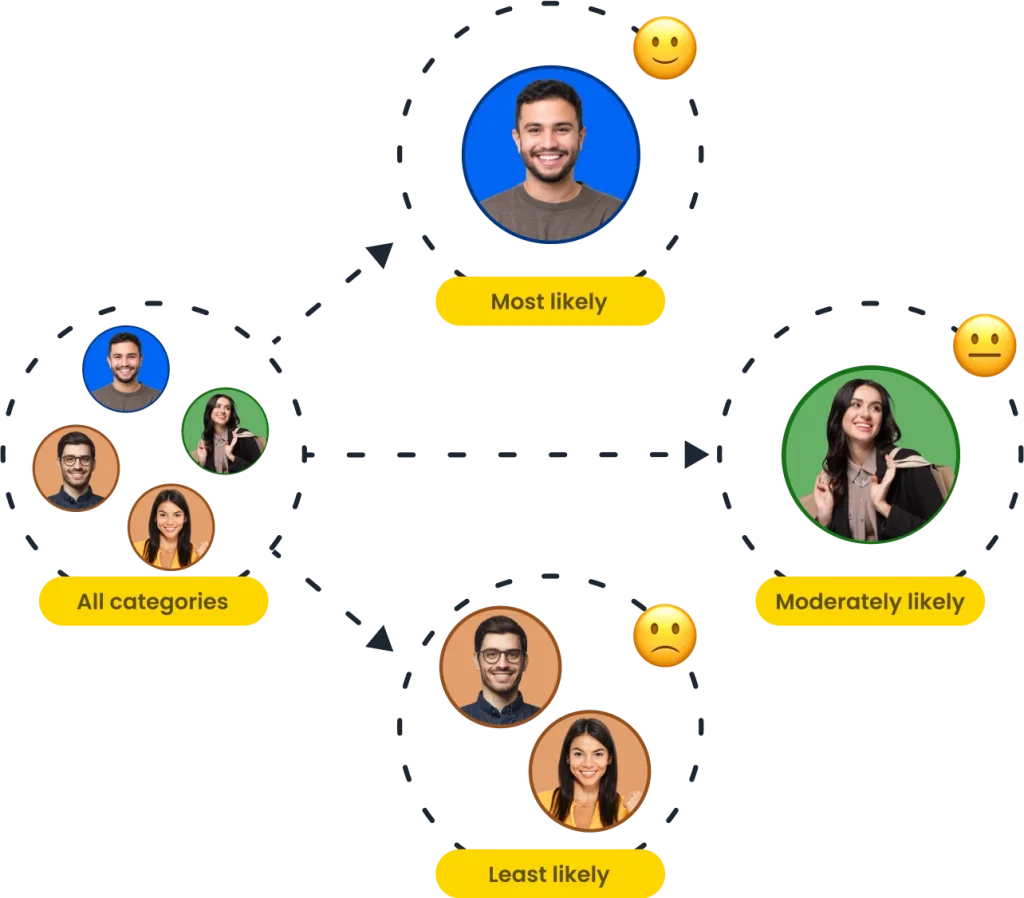
Crack the Code to Engagement
Excellence with Predictive Segments
Precision targeting based on your users’ past interactions.
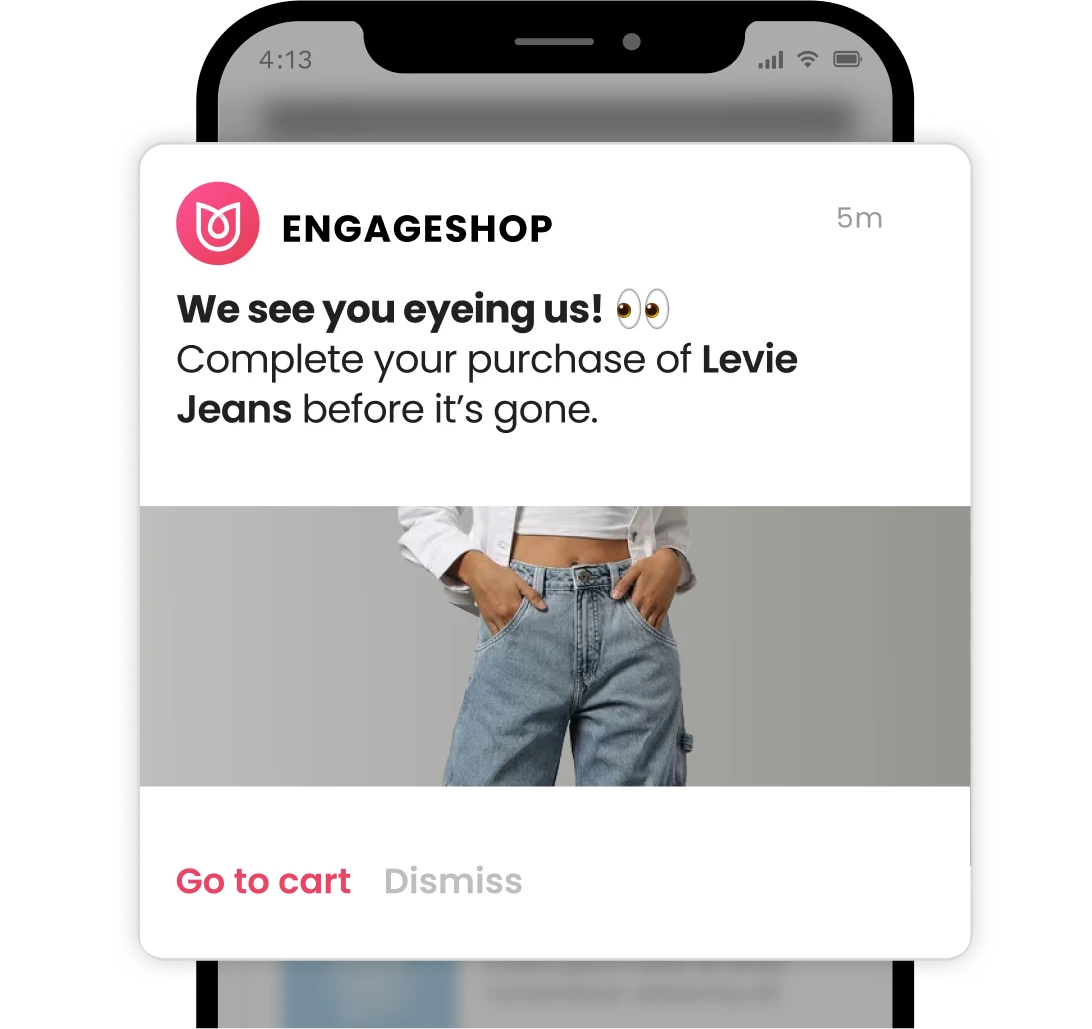
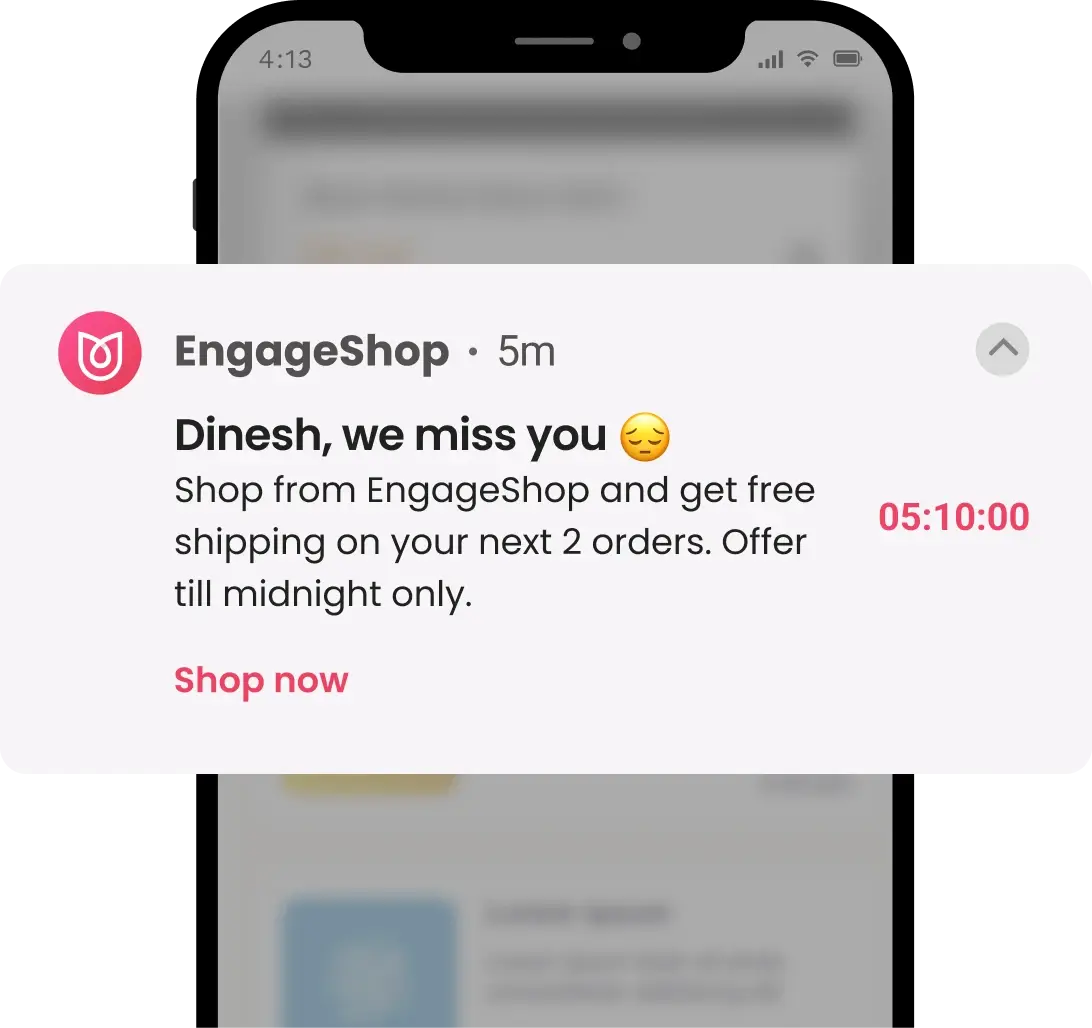
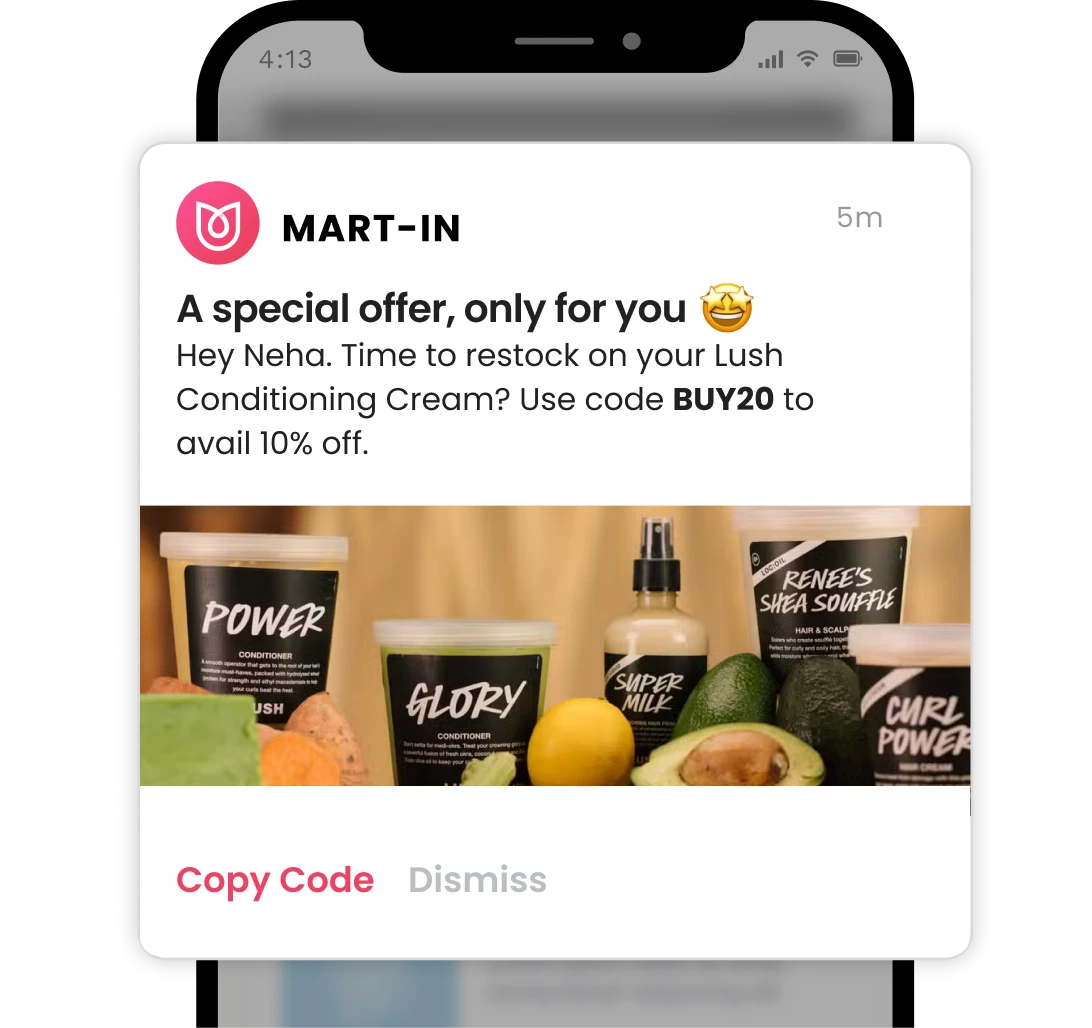
Turn clicks into cash
Pinpoint prospects primed to purchase, then seal the deal with tailored offers.
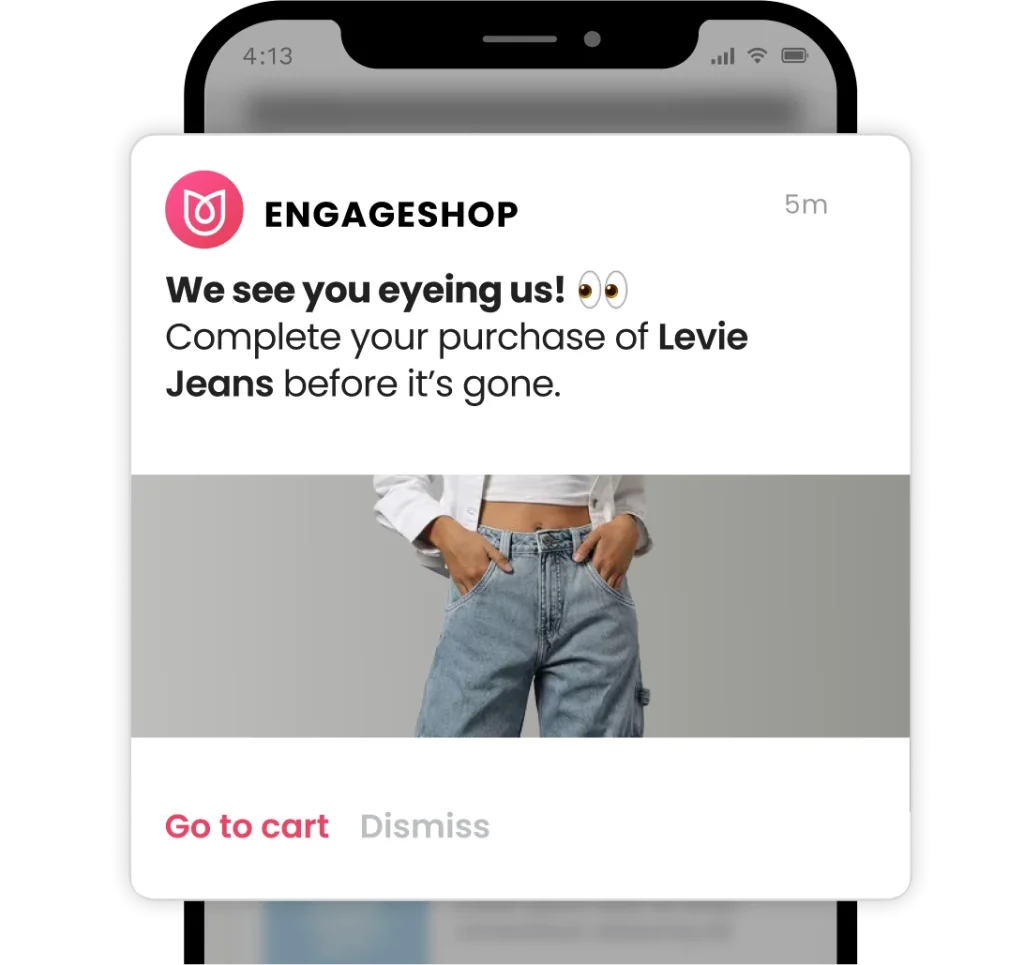
Churn no more
Spot and spoil potential escapers with irresistible incentives.
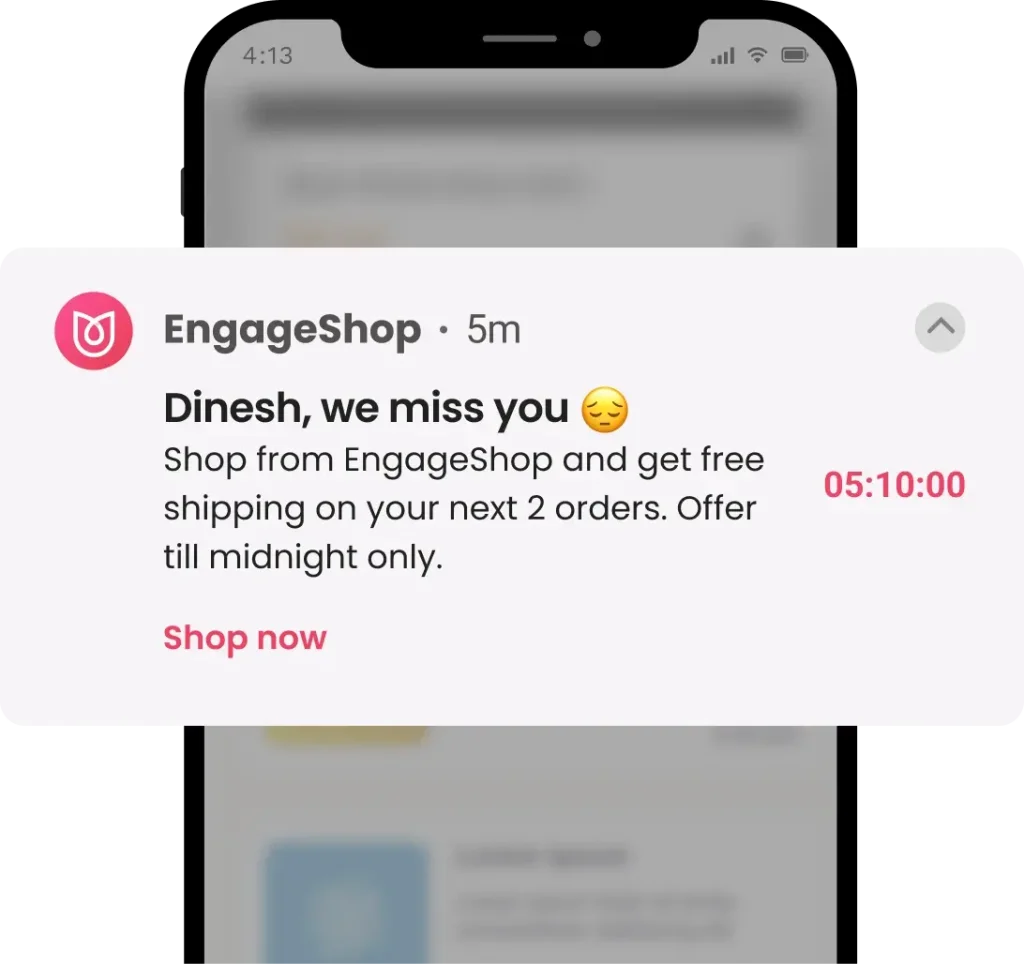
Promo perfection
Serve up discounts as unique as your customers' spending habits.
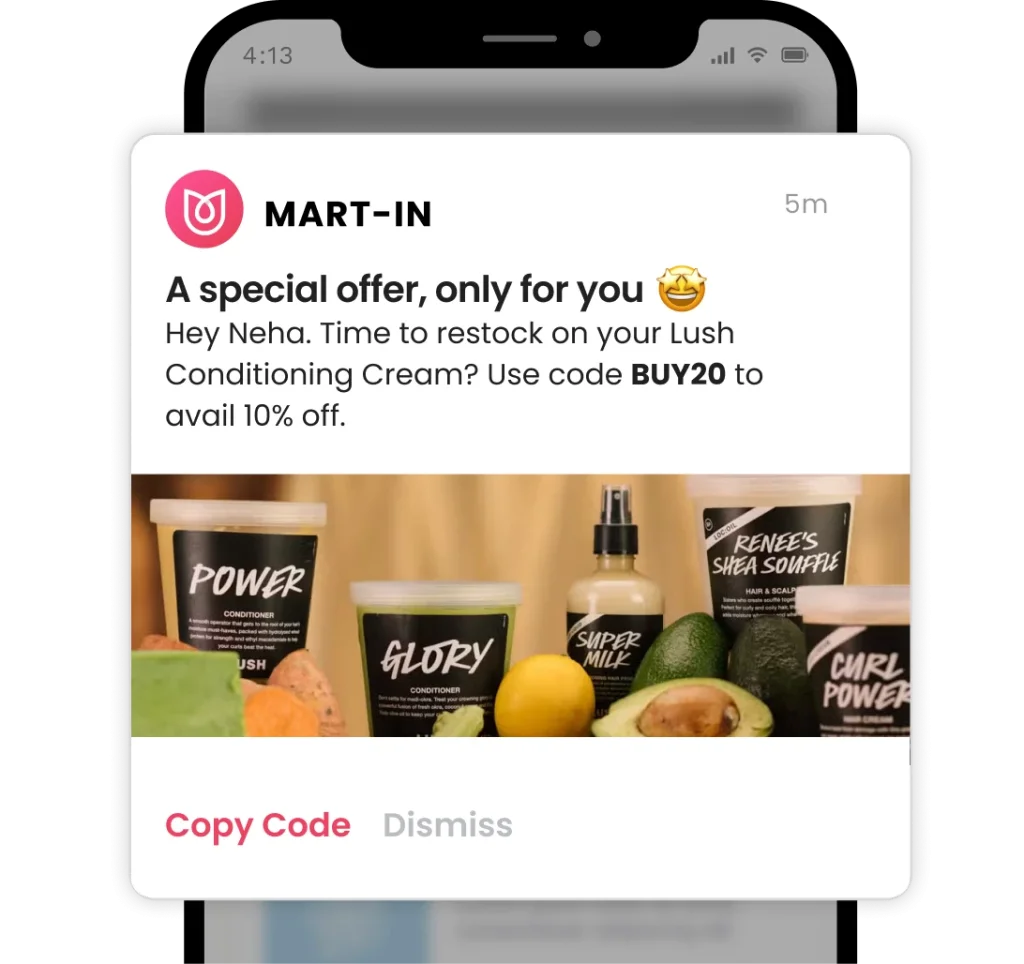
A word from our customers
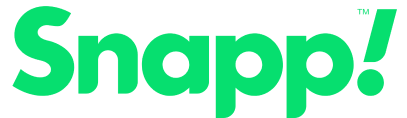
Increased their App re-installs by
45%
WebEngage has enabled us to create highly targeted campaigns by bringing customer data to the forefront

Muhammad Zohaib Anwaar
Group Director - CRM
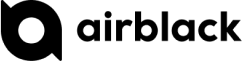
Increase in Subscription Rate by
13%
The WebEngage team is very consultative and helps us focus on the right things. Today we cannot imagine our life without WebEngage.

Videt Jaiswal,
Co-Founder, Airblack
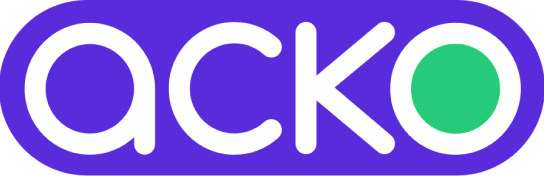
Increased their Policy Renewals by
17%
Win-back and policy renewal campaigns have contributed significantly to our overall revenue.
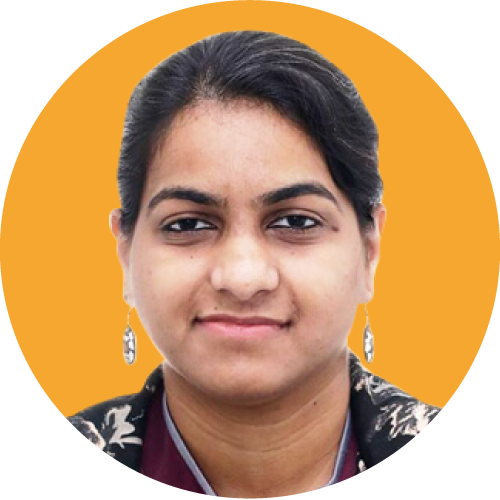
Payal Saxena
Associate Director - Digital Marketing
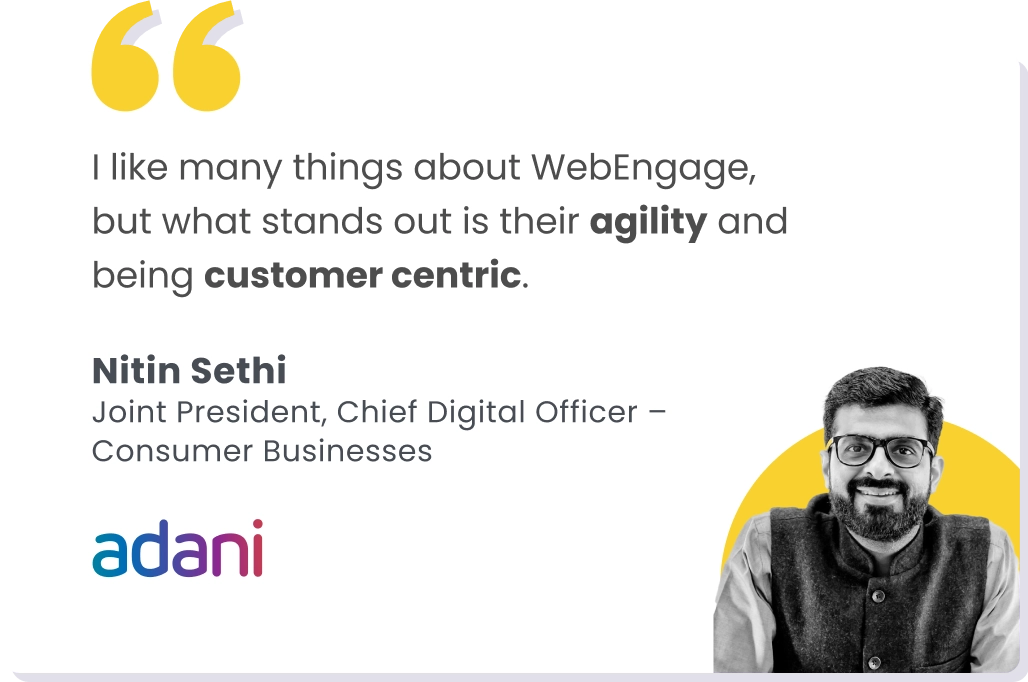
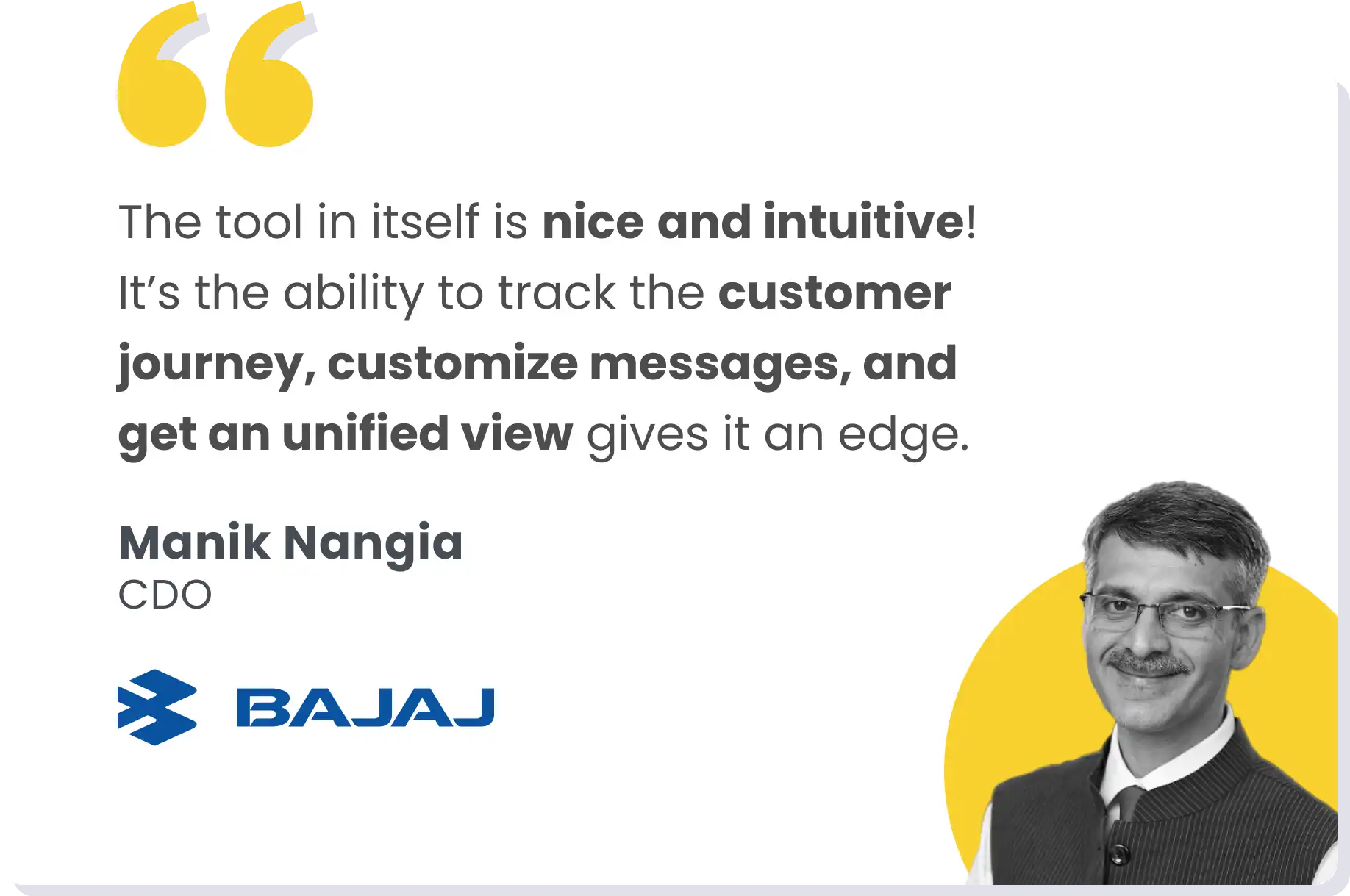
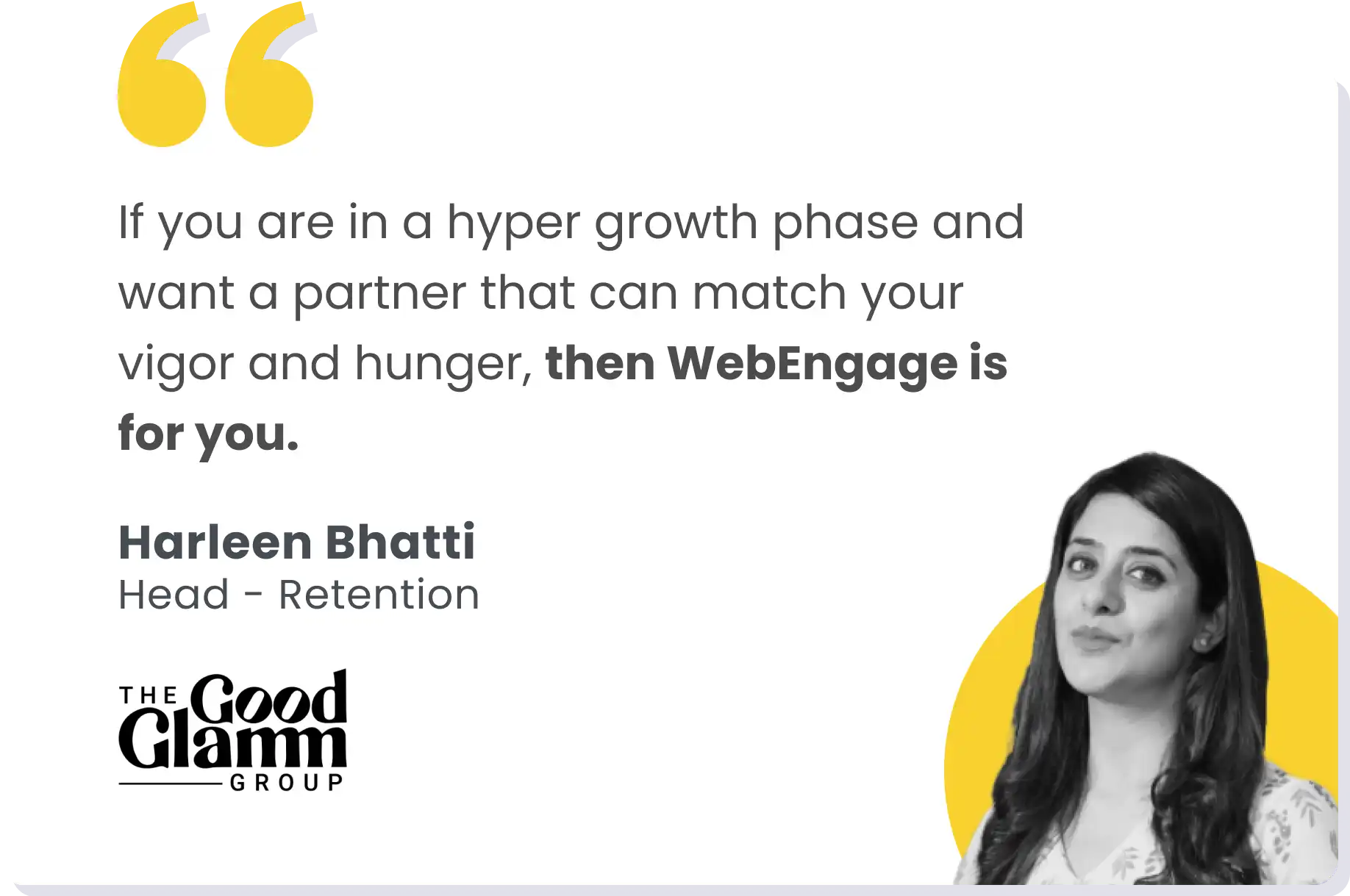
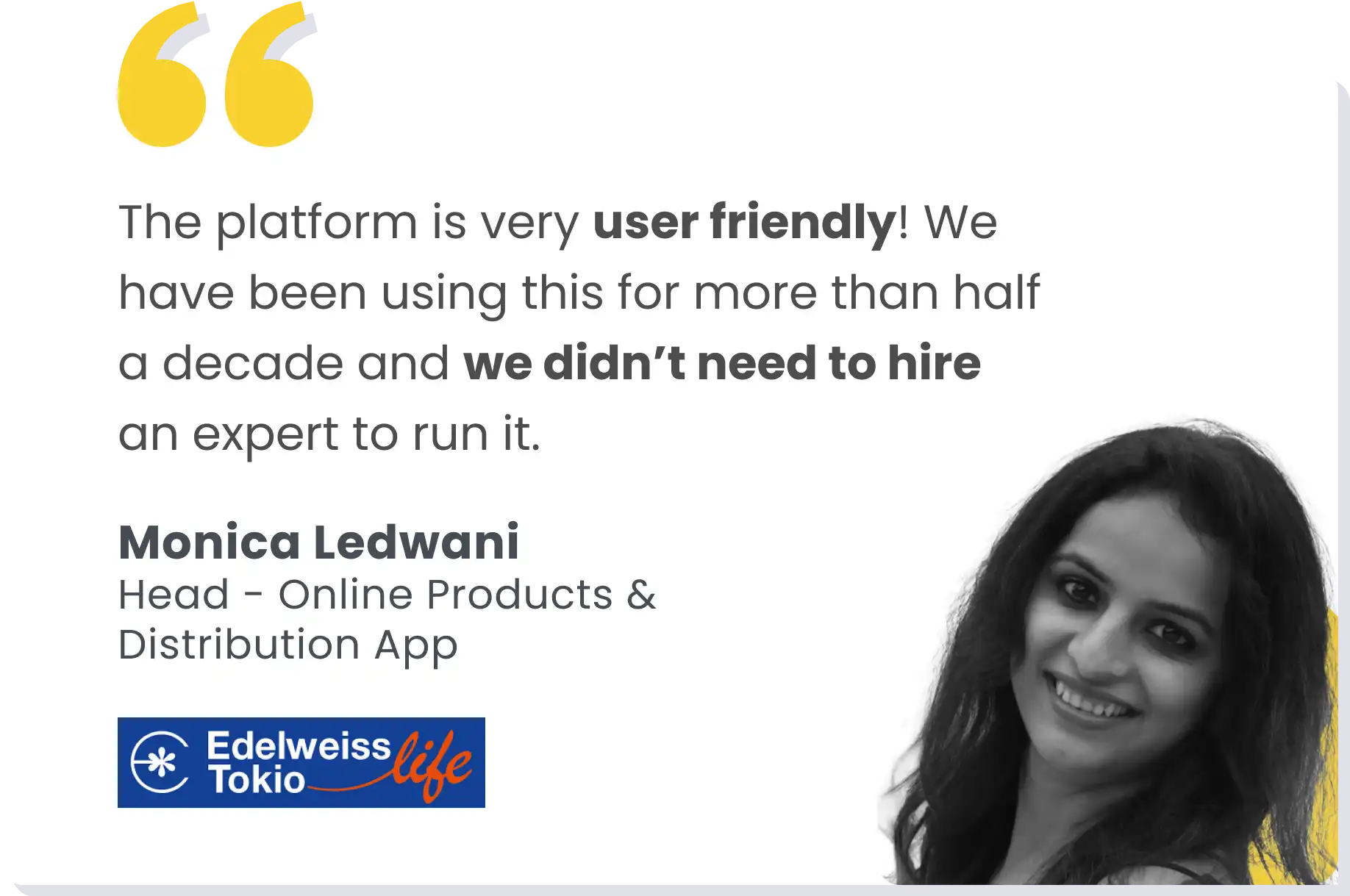
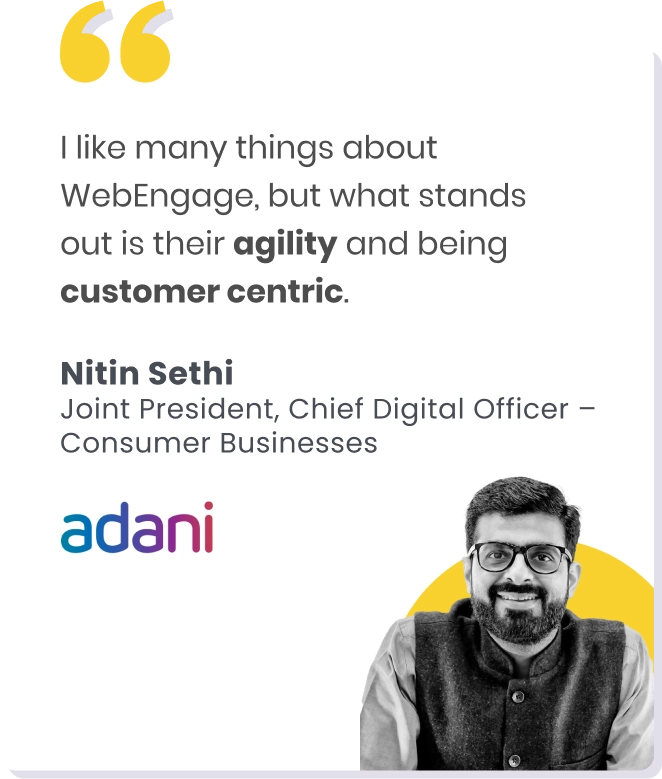
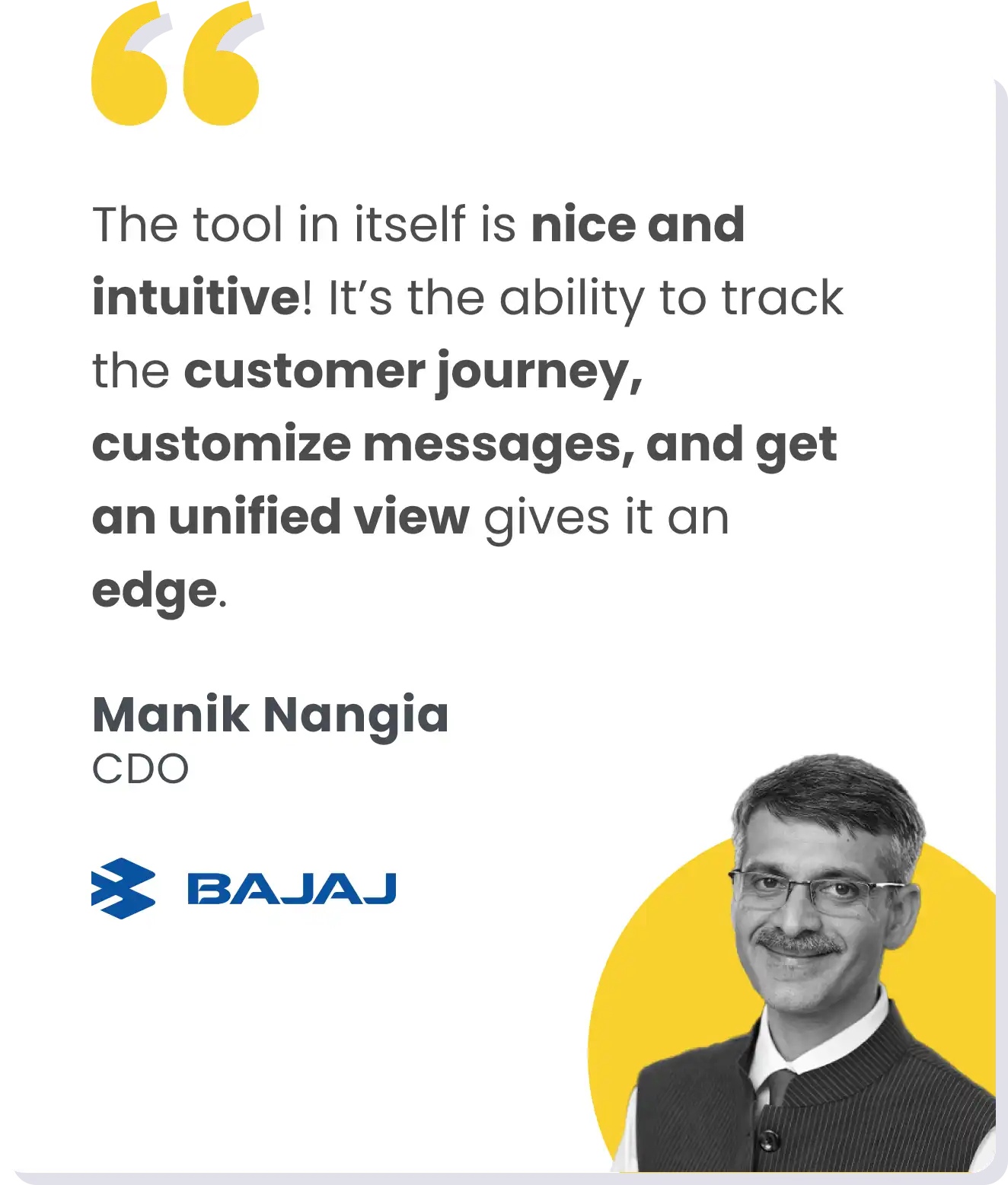
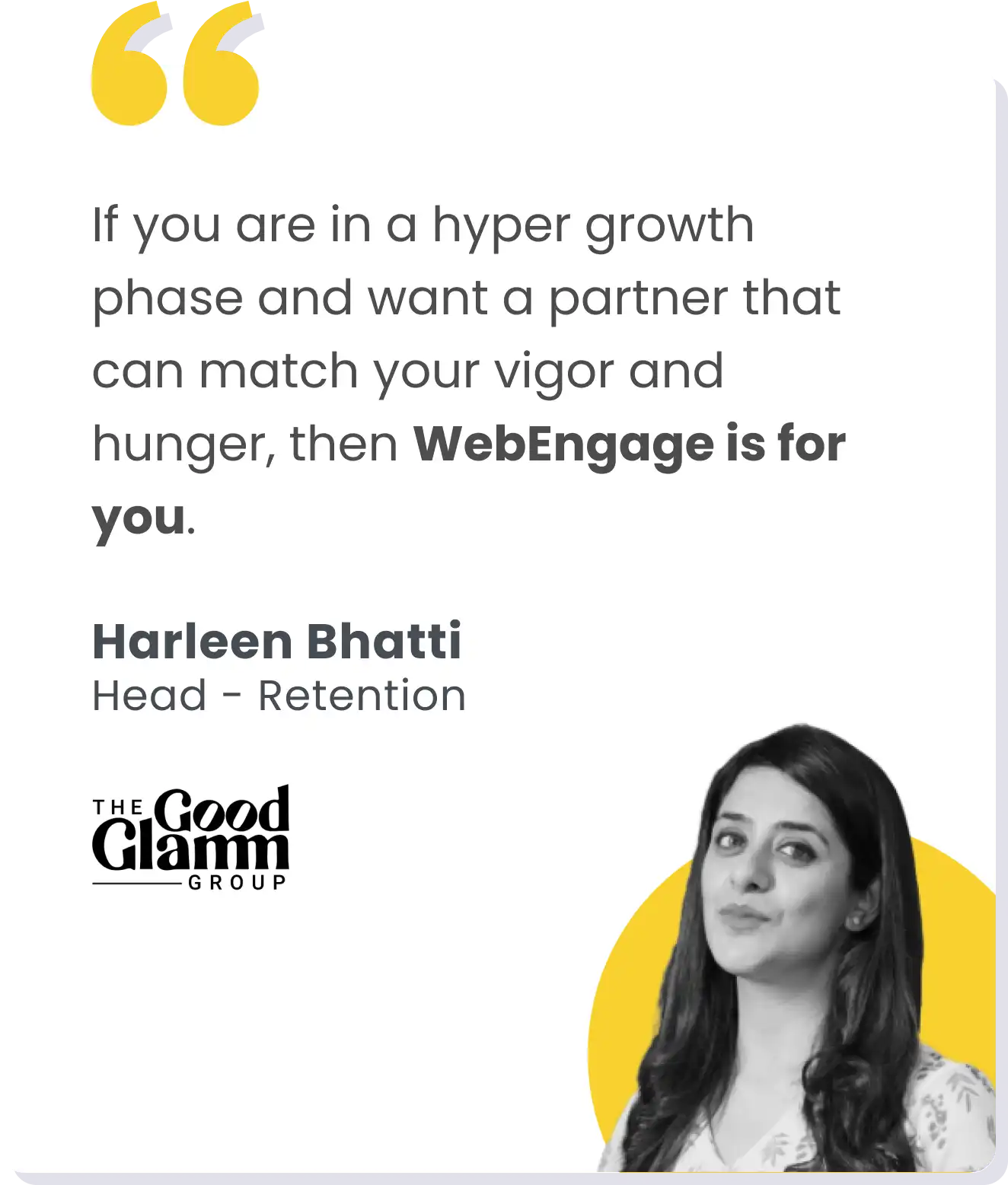
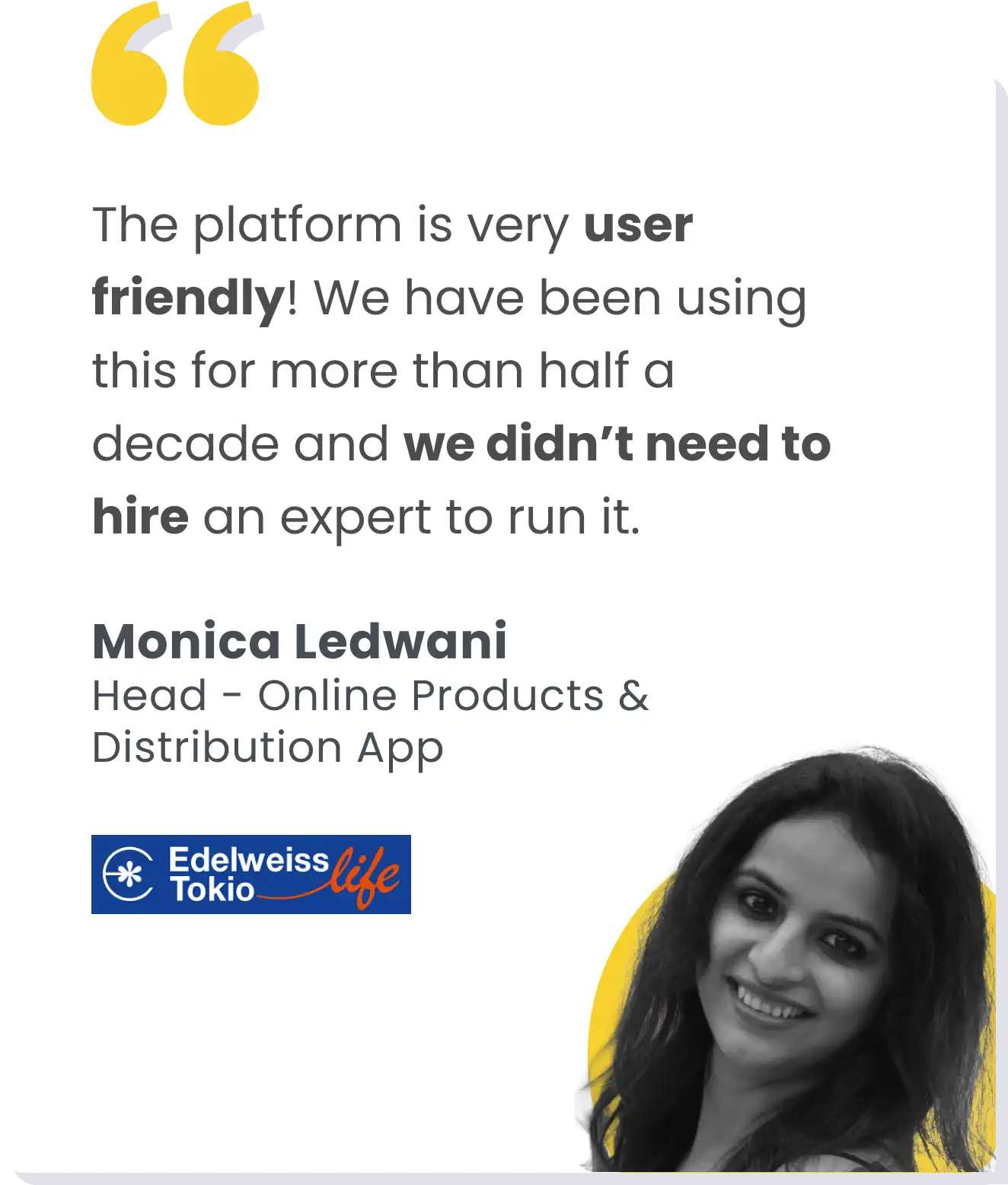
Trusted by 800+ Global Brands

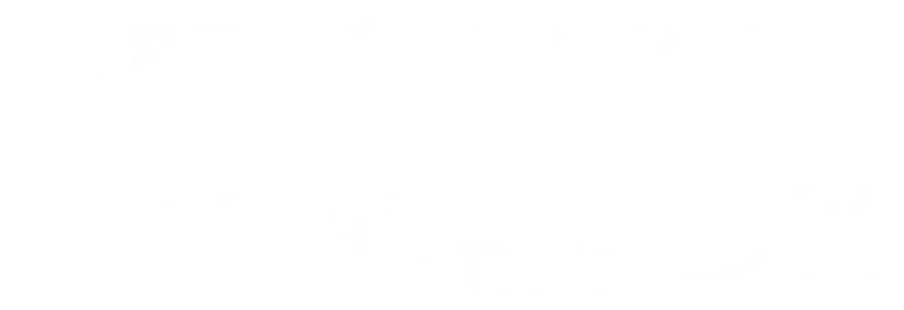