As travelers around the world wriggle out of a 3 year long hiatus, take our word that 2023 is going to be a year of travel-like no other. According to Booking.com, 68% people will go heavy on making travel plans in 2023. But an influx of enquiries implies that customers will do anything to get great deals.
If you’re running a travel and/or hospitality business, you know that a ‘good season’ means a lot of traffic, but it also brings poor conversion rates. The travel and hospitality industry has one of the highest cart abandonment rates at a whopping 85%. There could be many reasons for this – lower prices, better deals, lack of personalization, or poor loyalty programs.
76% of consumers get frustrated by businesses who do not offer personalized experiences. Users no longer have the time to endure cognitive overload and do the hard work of ‘searching’ for what they need, because what they need is understood by their favorite products (growth owners). What they seek is nudges from the brand that makes their experience easier.
We call these nudges product recommendations – a handpicked curation of suggestions over services created using machine learning and complex systems to offer a unique experience. These recommendations are created using a variety of inputs like location, past browsing history, current trends, offers etc.
Can product recommendations save the travel & hospitality industry?
79% of consumers expect brands to offer personalized digital experiences. So something as simple as allowing customers to pre-fill data in the search tab or remembering their most often typed destinations could come in handy while booking. Travel OTAs (online travel agency) are now also remembering the most commonly used filters as a hook to retain customers.
But how to ensure that users are seeing content and offer personalized for them? The answer is by using relevant data. You need to collect the right data from your customers to show them exactly what they want. User events can be captured in your backend and sent to your CRM platform to analyze your customers based on usage data and later cluster them into user properties to target.
The bigger question is, what kind of events should a travel and hospitality brand capture to better understand its customers? There are a few efficient ways to start with:
- User Demographic: User demographics such as age, gender, ethnicity, or income levels can significantly impact the performance of recommendation engines by understanding an ICP (ideal customer profile) at a closer, more personal level.
- Location preferences: A unique way to look at this would be making recommendations based on the most commonly visited destination from a specific location – for example suggesting beach destinations to people from a landlocked city or showing the most travelled destinations at a user’s current location level.
- Email: Closing the loop with customers is an incredibly potent tool from a personalization standpoint. When customers receive emails from brands, their expectations soar in terms of personalization.
- Frequency of travel: Building cohorts of users who have the same habits is a highly underrated way to make product recommendations.
Personalization done well at the scale
Booking.com is a great example of personalization done well at scale across many pages of the website. If you’ve ever made a reservation from booking, you’d know that the brand is sure to remember your history and allow those details to flow in for your overall experience.
Booking.com uses advanced machine learning to capture multiple data points; a user’s unique search history, location, preferences etc. The brand dissects users’ search history and booking patterns to understand their travel preferences.
For instance, if a user consistently books hotels with a gym or spa, Booking.com may recommend hotels with similar amenities in the future.
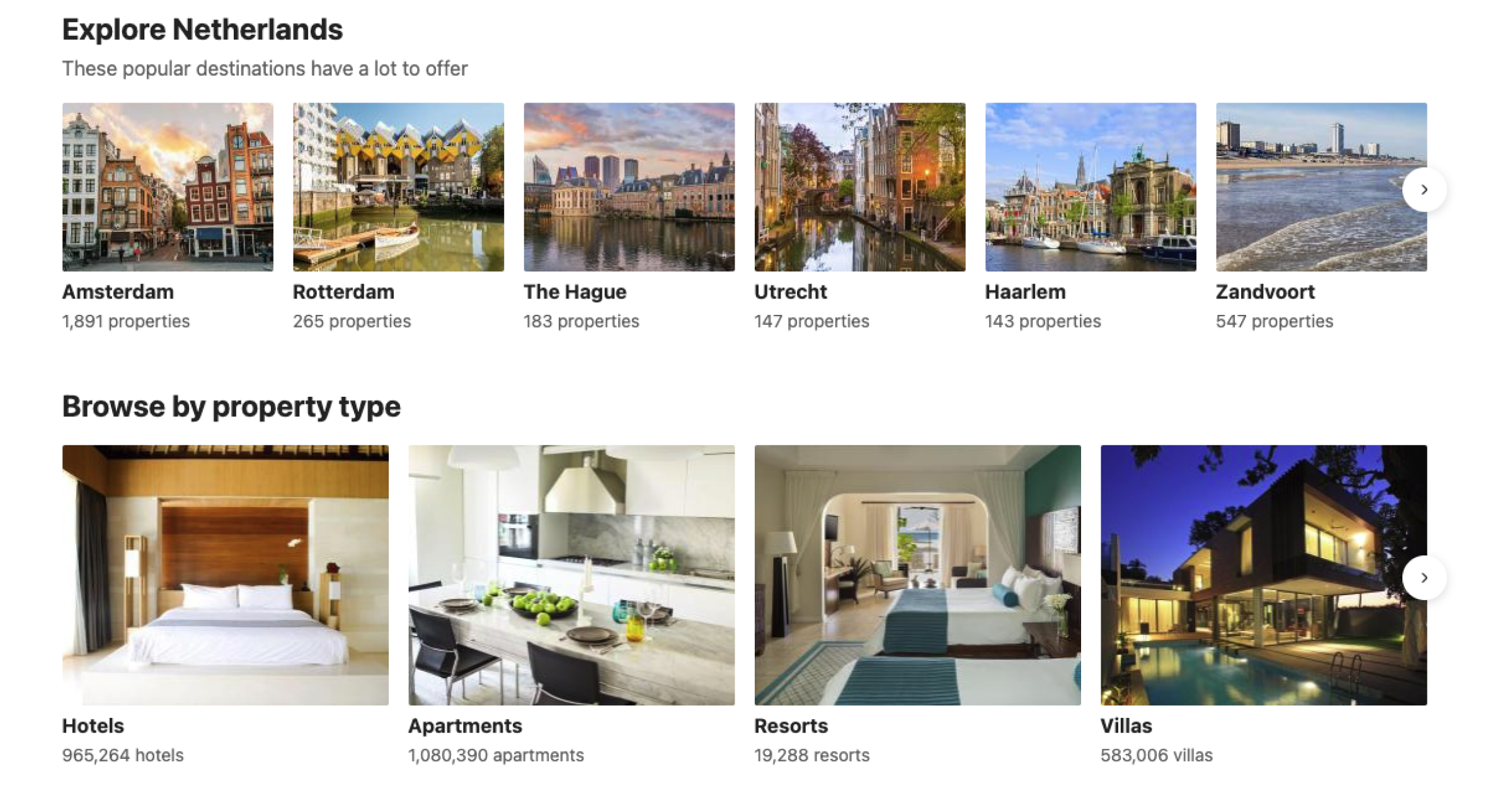
The brand famously relies heavily on feedback to identify common themes and areas that require improvement and then makes recommendations to the platform for an even better-personalized experience for its users.
The brand’s recommendation tab that sits underneath the search bar helps people with suggestions about their history, location, IP details, segments etc.
76% of consumers get frustrated by businesses that do not offer personalized experiences.
At the most fundamental level, all sorts of useful info — search history, travel history, interactions with Booking.com’s User Interface, etc. — are fed into ML models. This data is then analyzed and used at multiple levels for enhancing the user experience.
Every day, nearly half a trillion events are processed, and the more information the models gather, the smarter they become about making associations between different locations, user types, product types to provide product recommendations. Thus providing the hyper-personalized ‘Home Page’ we all love.
The foundation of travel and hospitality recommendation models
- Destination-centric recommendation engines
- Location-centric Product Recommendation Engines
Top 10 product recommendations for the travel and hospitality industry
- Geo-targeting local discounts: Geo-targeting means marketing to a set of specific users based on their location. The more relevant an ad is to a user, the more likely they are to convert. You can track the current location of your travelers and offer discounts and offers according to the local attractions and services. Here Agoda.com shows a full list of activities to do while in Goa (after detecting the location).
- Geo-targeting store locations: Brands can also recommend to users the top shopping or souvenir stores based on their current vacation destination and save them both time and effort. You can leverage physical store locations to attract nearby customers. Here, Agoda.com offers a Food Walking Tour and Jaipur Bazaar as part of their Jaipur ‘Activity Package’.
- Holiday-based: People make extravagant travel plans during the holiday season, and you can build your recommendation engine in a way that it curates travel recommendations according to a specific holiday.
- Post-purchase: Your recommendation engine doesn’t end during or before purchase, it has to continue post-purchase as well in the form of feedback, thank you notes, and upcoming holiday recommendations via SMS, Email etc. We talked about Airbnb building their experience page that populates once you book a place – now how incredible is that recommendation?
- Season-based: To satisfy travelers’ demand across seasons, a rather popular format is offering recommendations based on the general mood.
- Off-season: This can be an effective recommendation for a lot of travelers who like to travel during the off-season to avoid the rush.
- Peak season: Your recommendation engine can curate special peak season recommendations during summers and winters when most people plan a vacation.
- Big events based: You can also curate special recommendations on upcoming big events based on the location of a user. Example: recommending special St. Patrick’s Day packages to people whose current location may be Ireland.
- Returning Customer: This is a crucial cohort to target because if a customer is back on your product then they probably saw something of value. You should always have a separate reactivation or welcome back campaign for this cohort and your product recommendations should be catered to their behavior when they were last active.
- Loyalty and Rewards: Based on what stage of growth your product is in, you can build a tiered loyalty program to instill brand loyalty amongst your users. This helps in building a community for your customer base.
- BNPL recommendations: Travel can be a costly affair, which is why offering BNPL (Buy Now Pay Later) services is a great way to ease the burden on your customers. It is crucial to prominently showcase this service at various stages on your website. This will help you drive your Engagement and Retention campaigns by adding BNPL paying options if a user abandons their cart.
- AI-powered Chatbots: Making travel bookings can be exhausting – from where to stay to how to commute – travelers are swamped with a lot of choices to make. In such a case, an AI-powered chatbot can aid the need. In the travel industry, AI helps in recommending stay options, or things to do in the area.
Factors to consider
- User behavior data: 80% of business leaders report an increase in consumer spending by an average of 38% when their experience is personalized. This is the first and most crucial step in building a recommendation engine. The data can be garnered by two means: implicitly and explicitly.
- Here’s an example of an implicit mode of data collection from Booking.com.
- User Intent: Understanding the user intent through heat maps, search keywords, page traffic, etc., before building an engine is not only crucial but imperative. Be cognizant of what your user is seeking before you push.
- Personalization: Younger consumers are the most likely to react negatively after an impersonal experience. Make sure your recommendation engine solves for personalization and it is a core principle in the PRD (product requirements document) before building it.
- Contextual Relevance: Ensure your recommendation engine functions by providing contextual suggestions to your customers. It should optimize for seasonality so users can be rid of the cognitive load of searching for a specific occasion. Example: Christmas special discounts tickets & flights during Feb-March.
Mistakes to avoid
- Issues with data quality: One of the frequent errors is not giving sufficient attention to data quality, which can result in biased or inaccurate recommendations, leading to a subpar user experience. To mitigate this, it is crucial to ensure that the data is relevant, complete, and free of errors and is updated periodically.
- Lack of diversity: The problem of “echo chambers” is often experienced in recommender systems where users are only recommended items that they have previously liked. To prevent this, it’s vital to include diversity in the recommendation process by considering multiple factors like novelty and popularity.
Conclusion
There are strong indications that much like E-commerce, loyalty is a hard nut to crack in the travel, and hospitality industry. It is mostly the service, offer, and cheap prices that drive the transaction. And although such patterns create a rather challenging environment to operate in, many top companies have found refuge under product recommendations. Moreover, product recommendations can be the factor that can make or break your marketing strategies.
From increasing the stickiness of the user to increasing the average order value, all top OTA brands in the world have some of the other engines working to empower users’ experience and motivate them to transact. WebEngage offers a suite of levers for young brands to create customized, and highly personalized experiences.
Want to get started with a recommendation engine for your Travel and Hospitality platform? Speak to our experts and request a demo today!